Controlled oscillator¶
The controlled oscillator is an oscillator with an extra input that controls the frequency of the oscillation.
To implement a basic oscillator, we would use a neural ensemble of two dimensions that has the following dynamics:
where the frequency of oscillation is \(\omega \over {2 \pi}\) Hz.
We need the neurons to represent three variables, \(x_0\), \(x_1\), and \(\omega\). According the the dynamics principle of the NEF, in order to implement some particular dynamics, we need to convert this dynamics equation into a feedback function:
where \(\tau\) is the post-synaptic time constant of the feedback connection.
In this case, the feedback function to be computed is
Since the neural ensemble represents all three variables but the dynamics only affects the first two (\(x_0\), \(x_1\)), we need the feedback function to not affect that last variable. We do this by adding a zero to the feedback function.
We also generally want to keep the ranges of variables represented within an ensemble to be approximately the same. In this case, if \(x_0\) and \(x_1\) are between -1 and 1, \(\omega\) will also be between -1 and 1, giving a frequency range of \(-1 \over {2 \pi}\) to \(1 \over {2 \pi}\). To increase this range, we introduce a scaling factor to \(\omega\) called \(\omega_{max}\).
[1]:
%matplotlib inline
import matplotlib.pyplot as plt
import nengo
from nengo.processes import Piecewise
Step 1: Create the network¶
[2]:
tau = 0.1 # Post-synaptic time constant for feedback
w_max = 10 # Maximum frequency is w_max/(2*pi)
model = nengo.Network(label='Controlled Oscillator')
with model:
# The ensemble for the oscillator
oscillator = nengo.Ensemble(500, dimensions=3, radius=1.7)
# The feedback connection
def feedback(x):
x0, x1, w = x # These are the three variables stored in the ensemble
return x0 + w * w_max * tau * x1, x1 - w * w_max * tau * x0, 0
nengo.Connection(oscillator, oscillator, function=feedback, synapse=tau)
# The ensemble for controlling the speed of oscillation
frequency = nengo.Ensemble(100, dimensions=1)
nengo.Connection(frequency, oscillator[2])
Step 2: Create the input¶
[3]:
with model:
# We need a quick input at the beginning to start the oscillator
initial = nengo.Node(Piecewise({0: [1, 0, 0], 0.15: [0, 0, 0]}))
nengo.Connection(initial, oscillator)
# Vary the speed over time
input_frequency = nengo.Node(
Piecewise({
0: 1,
1: 0.5,
2: 0,
3: -0.5,
4: -1
}))
nengo.Connection(input_frequency, frequency)
Step 3: Add Probes¶
[4]:
with model:
# Indicate which values to record
oscillator_probe = nengo.Probe(oscillator, synapse=0.03)
Step 4: Run the Model¶
[5]:
with nengo.Simulator(model) as sim:
sim.run(5)
Step 5: Plot the Results¶
[6]:
plt.figure()
plt.plot(sim.trange(), sim.data[oscillator_probe])
plt.xlabel('Time (s)')
plt.legend(['$x_0$', '$x_1$', r'$\omega$']);
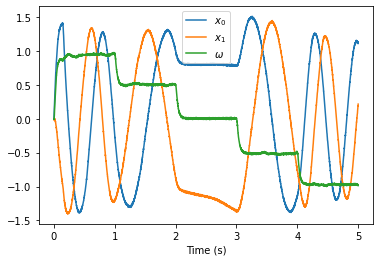